- Foundations of Deep Learning Models: Exploring the core principles and advancements in deep learning models (e.g., LLMs and diffusion models).
- Interdisciplinary Applications: Applying machine learning techniques across different domains by leveraging REAL data (e.g., healthcare, computational neuroscience, earth systems, and solar physics).
- Spatio-Temporal Forecasting: Predicting future events based on spatial and temporal data.
- Causal Discovery: Identifying cause-and-effect relationships within data.
- Interpretability and Scalability: Enhancing the transparency and efficiency of machine learning models.
Recent Projects
Full publications can be found from here.
We are grateful for the support of our sponsors:
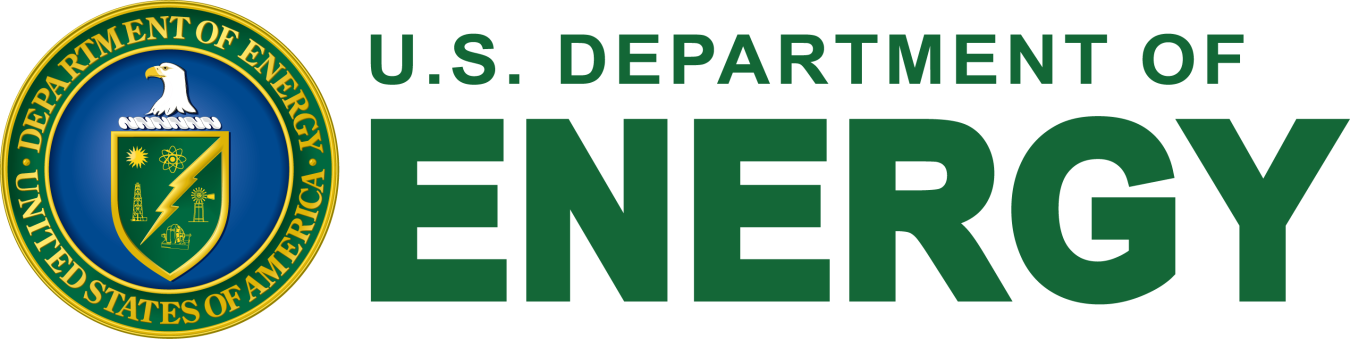
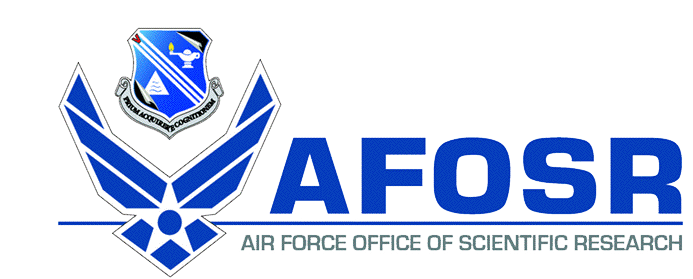
